How Machine Learning Is Changing the Game for Content Metadata
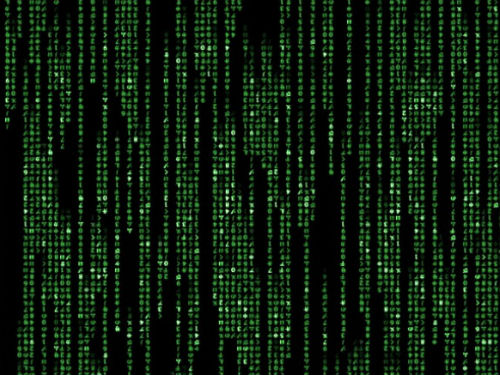
These are the best of times for entertainment content owners and distributors—but they are also very challenging times. There is more content—often great content—than ever before and also vastly more competition due to the rise of streaming services, as well as on-demand options.
Read More: Complete coverage of #CES2018
This presents a challenge for content owners and distributors: how to stand out from the crowd and help viewers find what they want.
Awash in all that content—not just professionally produced long-form content, but also highly viral digital-first content—viewers have a hard time wading through it all. In fact, it would take a single viewer more than 5 million years to watch the amount of video that crosses global IP networks each month, according to a recent Cisco Systems report.
That’s why it’s imperative for content owners and distributors to make it easy for viewers to search and discover their content. But to do that, they really need to know precisely what’s in their content. And to do that they need better content metadata.
Content metadata is the descriptive, image-rich programming information, such as title, actors, description, release date, running time, and genre. High-quality metadata is quite valuable because it can improve content discovery for consumers and make it easier for people to connect to the entertainment they find most informative and enjoyable at any given moment.
Discoverability is key
The rapidly evolving media landscape compels content owners and distributors to understand and define their content metadata at a much deeper and granular level than before. That’s because viewers demand quicker and easier ways to engage with content wherever and whenever they want. For distributors, this requires a new approach to creating content metadata and delivering personalized experiences for each and every viewer.
This is where machine learning comes in. Not only does machine learning help companies keep up with the tsunami of content, it can better enrich metadata and enable distributors to get the right entertainment in front of the right viewers at the right time.
Machine learning takes metadata beyond cast, title and descriptions, and enables content to be enhanced with many new data descriptors such as keywords, dynamic popularity ratings, and moods, to name a few.
The beauty of machine learning-powered metadata is that it can help surface the most relevant content in real time at just the right moment. In the old paradigm, viewers would rely solely on the most basic metadata, such as title and cast, to discover content. But with enriched metadata they can now search content by tone or trends or location—or numerous other categorizations that simply were not possible before.
Programming works better in real time
Better metadata can also help both online and traditional content distributors create more accurate and useful scheduling models. In the conventional TV programming model, networks need to know what they’re going to put on the screen weeks in advance. But that’s a limitation in today’s environment, where a programmer has no idea what may be popular two weeks from now.
Take a situation where a major YouTube star like Lilly Singh releases a new video that instantly goes viral. Most distributors can’t get that content up on their platform fast enough, so they miss out on millions of views. But machine learning can dynamically recognize that the video is trending in real-time and identify this to be served up to the distributor’s subscribers and help ensure that a service doesn’t miss out on that huge hit.
Traditional media work flows will also benefit from machine learning via the algorithmic matching of metadata to ever increasing content line-ups, enabling the content to get into distributor systems exponentially faster than what is possible via traditional methods.
Unlocking value for content creators
Content creators also face a set of new challenges, especially in a world where content is experiencing explosive growth. Now that consumers have more choices for content, one big question remains for content creators: How does their content get discovered and enjoyed?
That content could be their current “traditional” long form content, their back catalog of content, or the new digital-first content they are creating. The challenge is that existing metadata typically only includes title, cast, and synopsis, and oftentimes the quality and accuracy of the content is lacking. The problem is even worse for back catalog content.
The value of machine learning is that it can analyze large amounts of unstructured information and determine the relevant data to map to large amounts of content, thus making that content more discoverable and viewable.
Here’s an example. A typical piece of content from a major studio typically has a limited set of metadata. But what if you could analyze information from all over the Internet to add relevant data to that content? Things like theme, tone, mood, story, trending data, cross category links, entity relationships, etc.
This is no small task. Out of the huge volume of data on the Internet, how do you determine what is relevant to the content at hand? What’s more, data formats and structures are constantly changing such that you cannot simply write “rules” to identify what is relevant data to your content.
That’s why machine learning is so vital. It can analyze all this unstructured data to make sense of it and then enrich metadata for the content. As an analogy, imagine email spam. The nature of spam is continually changing, so the backend system needs to continually learn—and do so at massive scale—so that it can effectively filter out those junk emails.
Not people vs. machine, but people enhanced by machine
Machine learning certainly represents the future of metadata. But that doesn’t mean the need for human editors is going away. As content genres get more specific—such as “dysfunctional-family animated comedies” or “timeless Italian soap operas”—the need for human experts to curate this content also increases.
Think about how companies like Lyft, TaskRabbit and Postmates are able to leverage technology to bring together the workforce it needs at the location and exact time it needs, mapping workers to relevant tasks. The same principle holds true for editors of metadata. If you want your catalog of Spanish horror content to thrive, you’d prefer true aficionados of the genre editing that content, because they can do a much better job than anyone—or anything—else.
Machine learning can help find these experts at a massive scale and can also help edit their work. It has the ability to compare inputs from these editors and determine the consistency and accuracy of the data, resulting in the highest quality and most relevant metadata. At the same time, expert human editors can fine tune the initial data from machine learning techniques to bring unparalleled quality to the content metadata. You see this dynamic today where companies like Facebook and Google are combining people with machine learning to ensure appropriate content for their respective customers.
As the volume and breadth of content continues to explode, machine learning-powered metadata will enable speed at scale. It can be used to create better, more personalized entertainment experiences for viewers, while driving critical new revenue opportunities for content creators and distributors.
--Roz Ho is senior vice president and general manager, consumer and metadata, TiVo
Multichannel Newsletter
The smarter way to stay on top of the multichannel video marketplace. Sign up below.